Customers may not fully understand AI and machine learning technology yet, but channel partners have been building their expertise to make the use cases a reality for clients.
Whether channel partners or customers remain skeptical of the hype surrounding AI, the market is moving in full swing with improvements to AI and analytics technologies. This is paving the way for new applications, including real-time data analysis. This may interest you : MSPs: Microsoft Is Fastest Growing Partner In Managed Services ‘Golden Era’. It is also creating an opportunity for channel companies to help small businesses take advantage of them.
The current state of AI and data analytics
MSPs and consultants are listening to the request to automate with AI. On the same subject : Managed Security Services Provider (MSSP) News: 19 January 2022.
“Everyone wants automated reports or dashboards to see ‘how my business is doing’ and be able to look at key KPIs, ” said Ryan Ries, Practice Manager for Data Practice, Analytics and Machine Learning at the company. Consulting Mission, which launched a hands-on learning advisory machine a year ago and also offers AWS analytics and data skills.
The company has a couple of data scientists on staff who work on computer vision and predictive analytics projects and create customized machine learning models for clients, Ries said.
Jaret Chiles, Mission’s vice president of consulting services, said he doesn’t believe MSPs need to add AI and data analytics managed services to stay competitive right now.
“It’s a differentiator. It’s more vertically focused and a good business strategy depending on what you’re tackling,” Chiles said.
As data maturity across various industries has evolved over the past year, IT consulting firm Pythian has aligned its data services to both broaden capabilities and deepen the expertise offered, said CTO Paul Lewis.
“We have added skills for cognitive services, including data exploration, processing, model training, model evaluation, model implementation, online prediction and application of machine learning APIs. focused on the cloud, ”Lewis said.
Client challenges for partners with AI and data analytics projects
Partners face challenges in assisting clients with these types of projects. There is still “a giant educational piece about data and analytics. This may interest you : Managed services benefits ease enterprise IT security woes. People are being bombarded with ads saying” machine learning will solve all your problems. That’s not true, “Ries said.
He recalled talking to a client recently who didn’t understand the fundamentals of machine learning. Often times, boards will push for machine learning projects, but companies haven’t thought about their use case, Ries said.
“I’ve attended meetings where the client says, ‘My advice says I have to do this; tell me about other companies that are and why do I have to?'” They need to be able to articulate a problem they want to solve and be able to collect the data to solve that problem, he said.
In some cases, companies know the problem they want to solve, but haven’t collected the data. If they want to track historical trends, the data won’t be there.
The shortage of AI and analytics professionals to solve real-world business problems remains a major problem in 2022.
“Additionally, IT departments in small and midsize businesses typically don’t include experts who can use advanced data strategies such as governance, privacy, mobility and consumption,” Lewis said.
Both Ries and Chiles agree that finding talent is a challenge for SMEs and that there is now a huge demand for managed data analytics services.
“One of the things that [the] industry is trying to understand right now and struggling with is the lack of talent and … I need a full-time person to manage my dashboard solution. “Machine learning, is it ‘Do I? I have enough work for a full-time person to manage my machine learning operations pipeline and is my model kept up to date?'” said Ries.
This prompted Mission to develop a managed ML offering with the ability for customers to purchase a certain number of hours per month.
“Smart MSPs are investing in their existing staff by providing in-house training and access to certifications and other avenues of accreditation for professional development,” said Carolyn April, senior director of industry analytics at CompTIA. Vendors also recognize the value of partnering with MSPs by offering necessary technical training as part of their partner programs. “In the end it’s a win for both sides.”
MSPs cite two main barriers to adding emerging technologies and other premium services to their offerings: the need for technical training among staff and the difficulty of hiring people with the necessary skills. As of April, his research showed that 44% of organizations surveyed report a need for technical training and 37% report hiring difficulties.
“Skill gaps and hiring barriers are nothing new in the life of today’s tech entrepreneur, but as companies seek to elevate their game with services beyond the stakes, this problem will compound,” April said. . Skills are out there, but it’s a job-seeking market today, and MSPs are competing not just with other tech companies for new hires, but with companies from every industry, he said.
The ML/analytics market in 2022
As companies emerge from COVID-19, along with the Grand Resignations, Pythian customers seek help building new data capabilities and maturing their data strategy, Lewis said.
“More mature customers are engaging Pythian with advanced analytics projects, uncovering insights across large datasets, building new algorithms for demand forecasting or behavioral modeling, or simply adding scale to their data science practices to create operational technology processes.” , he has declared.
Dig Deeper on MSP technology services
DattoCon Now points to profit in SaaS defense, continuity
Why and how MSPs adopt cybersecurity industry standards
IT consultants go for green cloud computing
N-able looks to plug MSP marketing gaps
What are the 4 types of business analytics?
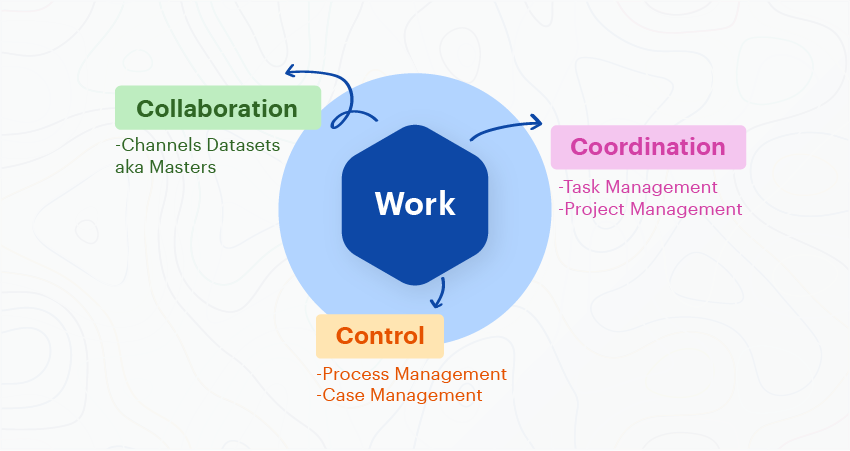
Beginner’s Guide to 4 Types of Analysis
- Descriptive analysis.
- Diagnostic analysis.
- Predictive analytics.
- Prescriptive analysis.
What is step 4 in the business analysis process? Step 4: Foresight and Optimization In today’s era, business analytics is all about being proactive. In this step, you will use forecasting techniques, such as neural networks or decision trees, to model the data.
What are types of business analytics?
There are three types of analytics that companies use to guide decision making; descriptive analyzes, which tell us what has already happened; predictive analytics, which shows us what could happen and, finally, prescriptive analytics, which informs us of what should happen in the future.
What is business analytics and example?
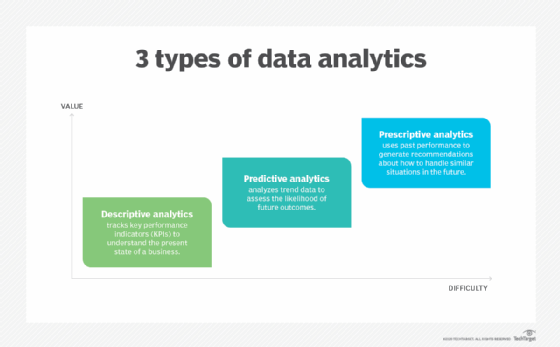
Some examples of business analytics include the operation and management of clinical information systems in the healthcare sector, monitoring player spending and developing casino loyalty efforts and streamlining fast food restaurants by monitoring customer peak hours and identifying when sure …
What is the significance of business analysis? In particular, business analysis refers to: Acquisition and processing of historical business data. Analyze that data to identify trends, patterns and root causes. Make data-driven business decisions based on that information.
How can analytics help a business example?
Business analytics can help you in supply chain management, inventory management, goal performance measurement, risk mitigation plans, improve efficiency based on product data, etc. For example: the manager wants information on the performance of a machine that has been used in the past 10 years.
What is a popular example of data analytics?
A real example of a company that uses big data analytics to increase customer loyalty is Coca-Cola. In the year 2015, Coca-Cola managed to strengthen its data strategy by building a digital-driven loyalty program. Coca-Cola’s director of data strategy was interviewed by ADMA’s chief editor.
How is analytics used in a business?
Business analysis, on the other hand, aims to inform changes in a company through the use of predictive models that provide detailed information on the outcome of the proposed changes. Business analytics uses big data, statistical analysis, and data visualization to implement organizational changes.
What type of data analytics is most difficult?
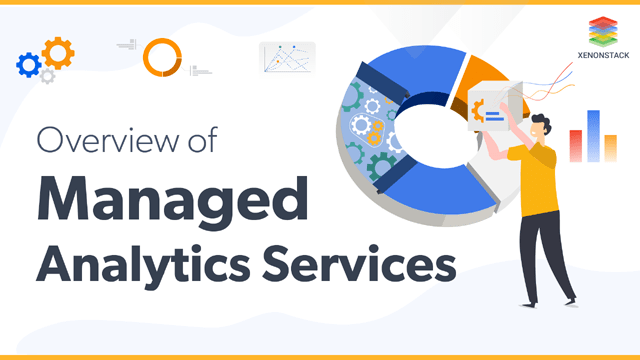
Prescriptive analysis is, without a doubt, the most complex type of analysis, involving algorithms, machine learning, statistical methods and computational modeling procedures. In essence, a prescriptive model considers all the possible decision-making models or paths that a company could take and their likely outcomes.
What is the hardest part of data analysis? The hardest part of data science is not building an accurate model or getting good, clean data, but defining workable problems and finding reasonable ways to measure solutions.
Is big data analytics hard?
As mentioned above, data analytics is not a difficult field to delve into because it is not highly academic and you can learn the required skills along the way. However, there is a wide variety of skills that you will need to master in order to do the job as a data analyst.
Does Big Data analytics need coding?
Learning to code is an essential skill in the big data analyst’s arsenal. You need to code to conduct numerical and statistical analyzes with huge datasets. Some of the languages you should invest time and money in learning are Python, R, Java, and C, among others.
Is Big Data analytics good?
Big Data Analytics has become critical as it helps improve business, decision making and provide the greatest advantage over the competition. This applies to both organizations and professionals in the Analytics domain.
What type of data analytics is more for optimization?
Prescriptive analytics, when used effectively, provide valuable insights for making the best possible data-driven decisions to optimize business performance.
Is optimization predictive or prescriptive analytics?
The key difference is that predictive analytics simply interprets trends, while prescriptive analytics uses heuristic-based automation (rules) and optimization modeling to determine how best to follow.
Which type of data is used for predictive analytics?
Predictive analytics uses historical data to predict future events. Typically, historical data is used to build a mathematical model that captures important trends. This predictive model is then used on current data to predict what will happen next or to suggest actions to be taken for optimal results.
Is it hard to learn data analytics?
Data analysts rely on skills such as programming in R or Python, querying databases with SQL, and performing statistical analysis. While these skills can be challenging, it is entirely possible to learn them (and get a data analyst job) with the right mindset and action plan.
How do beginners learn data analytics?
Can I teach myself data Analytics?
From learning code using Python, to learning multivariable computing, to learning how to develop machine learning algorithms, it is now possible to become a competent and competitive data scientist without spending thousands of dollars or years of your time on a postgraduate degree. secondary, diploma or certificate.
Why do we need business analytics?
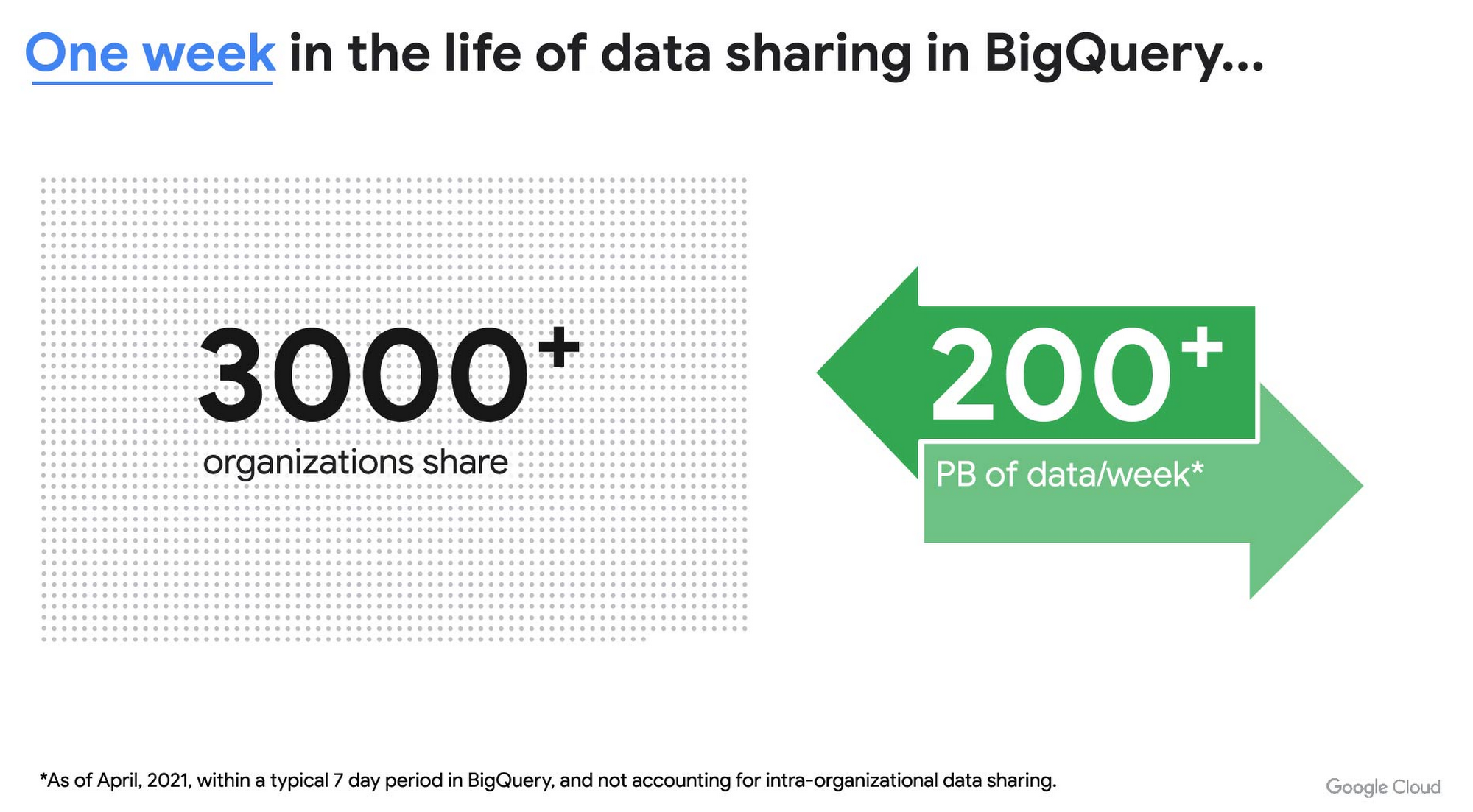
Business analytics can help companies make better, more informed decisions and achieve a variety of goals. By leveraging data, businesses can: Better understand consumer behavior. Get information about their competitors.
How is business analytics used?
Business analytics offers companies a way to plan for the future. By modeling trends in a company’s sales, profits, and other key metrics, these indicators can be projected into the future.
How is business analytics used in real life? Business analysts use various analytical techniques and approaches to improve financially relevant problems such as fraud detection, risk mitigation, product pricing, marketing campaign optimization, financial planning, forecasting, etc. These problems can be checked by a business analyst.
What are the 3 types of business analytics?
There are three types of analytics that companies use to guide decision making; descriptive analyzes, which tell us what has already happened; predictive analytics, which shows us what could happen and, finally, prescriptive analytics, which informs us of what should happen in the future.
What are the three common types of data analytics?
The three dominant types of analytics – descriptive, predictive, and prescriptive analytics – are interconnected solutions that help businesses get the most out of their big data. Each of these types of analysis offers a different view.
What is business analytics model?
Simply put, a business analytics model outlines the steps a company takes to complete a specific process, such as ordering a product or entering a new hire. Process modeling (or mapping) is critical to improving process efficiency, training, and even compliance with industry regulations.
What is the main goal of a business analytics model? Business analysis, on the other hand, aims to inform changes in a company through the use of predictive models that provide detailed information on the outcome of the proposed changes. Business analytics uses big data, statistical analysis, and data visualization to implement organizational changes.
What is an analytics model?
An analytical model is quantitative in nature and is used to answer a specific question or to make a specific design decision. Different analytical models are used to address different aspects of the system, such as its performance, reliability or mass properties.
What are the key components of an analytics model?
This article will discuss 4 main components of an analysis model, namely: 1) Data component, 2) Algorithm component, 3) Real world component, and 4) Ethical component. Knowledge of data science training courses is required to acquire skills in Components 1 and 2 (Data Component and Algorithm Component).
What are different analytics models?
There are four types of analysis, descriptive, diagnostic, predictive, and prescriptive.
What are the 3 types of business analytics?
There are three types of analytics that companies use to guide decision making; descriptive analyzes, which tell us what has already happened; predictive analytics, which shows us what could happen and, finally, prescriptive analytics, which informs us of what should happen in the future.
What are the three common types of data analytics?
The three dominant types of analytics – descriptive, predictive, and prescriptive analytics – are interconnected solutions that help businesses get the most out of their big data. Each of these types of analysis offers a different view.